PROTEIN AND PRODUCE FOOD NETWORKS: 16 ESSENTIAL CAPABILITIES
Maintain Business Continuity, Resilience and Operational Readiness Under All Conditions with a Focus on Customer Service Levels, Product Quality, and Least Landed Cost
2020 has been a year like no other for the food-related industries. With shifts in demand, shifts in supply, and dramatic changes in consumer behavior, many of these changes will remain permanent. How should companies respond, and how can technology help maximize your success in this “next normal” environment? In this paper, we describe essential requirements to make it possible. Our view is that when planning and execution operate together with real-time information, businesses are best positioned react to any variance and secure replenishment, while coordinating upstream supply for the highest customer service levels at the lowest landed cost.
Before the pandemic, food-based consumer spending was in somewhat of a comfort zone with 4% year-over-year growth on average over the past few years. At One Network Enterprises we have the ability to analyze aggregate market data from our install base for thousands of companies across the Food Retailing, Restaurant, and Food Service industries, along the full upstream network that replenishes those supplies. In fact, we move nearly a third of the food in the US today across our network. This 4% was fairly evenly split between retail food outlets and food service outlets which include restaurants, hotels, schools, hospitals, and prisons. This split was also reflected in share-of-wallet, which was also approximately 50%.
The Pandemic, Pantry Loading, and the Demand Shift
Once the pandemic hit and conditions changed, along with the associated restrictions, we witnessed an immediate shift in our network channel volumes. As many of us experienced when shopping at food retailers for our family provisions, the population shifted into “pantry loading” for certain consumables initially, and then later for longer term foodstuffs. Our customer networks experienced a 30%+ shift in food volumes from Food Service to Food Retailing which continued to grow as the pandemic deepened month over month.
The typical food retail supply network carries 65 days of inventory which exists to buffer the inefficiency and fragility present in supply replenishment, along with poor demand accuracy which compounds the problem. This results in only 96% in store/in stock availability on average and only 80% availability for promoted items. When running on One Network, these same environments only carry 25 days of inventory with 99% in-store/in-stock for both promoted and non-promoted items. The net is that for most retailers the pandemic flushed their excess 40 days of stock in 10 days due to pantry loading. These extra 40 days were there to protect them from deficiencies in their software, analytics, and processes — and without them they were fully exposed.
A network that runs with 25 days of inventory as opposed to 65 is designed to be both agile and responsive. Thus, it’s able to withstand most shocks, providing business continuity, resiliency, and operational readiness under most conditions. In this case, the bullwhip effect from the channel demand shift quickly disrupted upstream channels as well. Food packaging and processing became unbalanced with downstream demand and distribution channels unhinged, causing food to be trapped upstream with really nowhere to go. The amount of food entering the network had been being consumed by end customer demand through the previously balanced (but inefficient) system, thus any disruption would require alternate measures, given the same amount of food still needed to reach the population.
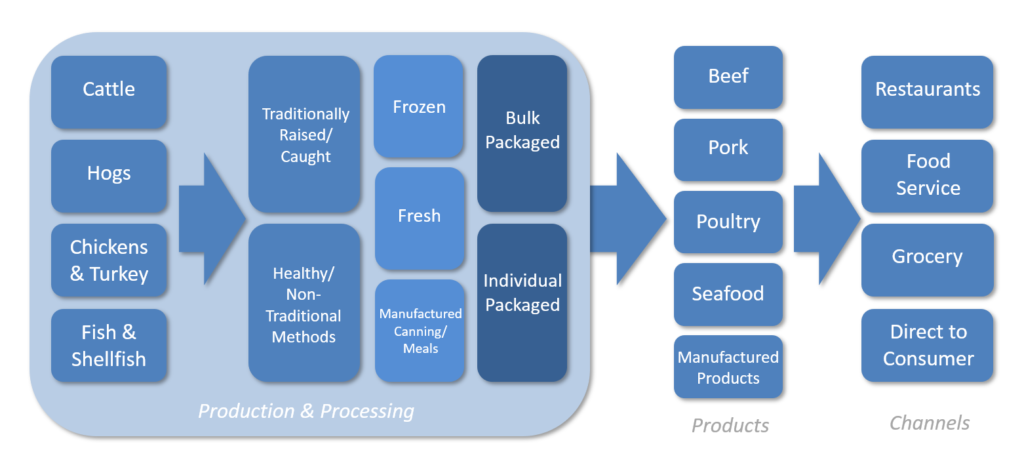
The Packaging Dilemma & Permanent Changes
The packaging issue between food service and food retail can be summed up as “bulk” vs “each’s.” For the most part, food service deals with bulk and retail deals with each’s, meaning individual items. It would take investment for bulk producers and packagers to switch to providing each’s. It becomes a question of risk in terms of whether the shift is mostly a medium-term event (with a smaller payback for the investment) or whether this is a more permanent shift (with a decent business opportunity upside). Considerations would include the flexibility to handle multiple order cancellations which would require upstream inventory to move through alternate channels, packaging options, and potentially include capabilities like micro-fulfillment centers and last mile delivery to ensure full consumption.
Forecasting demand scenarios in the coming months requires us to recognize that a certain percentage of the statistical shifts in product movement to the end consumer through new channels the food supply network is there to stay. This presents significant risk for food producers, packagers, and distributors, along with the food retailers and food service operators themselves. AI and machine learning will be key in understanding demand patterns in the “next normal” and drive increased demand forecast accuracy.
“Now, talking about results, One Network has allowed us to achieve best in class forecast accuracy levels, from the low 60s to the high 80s. We have essentially eliminated the bullwhip effect, and we have improved the efficiency of our inbound supply chain, reducing many, many of our suppliers’ costs.”
– Chief Supply Chain Officer, Global Restaurant Brand
What is the Bullwhip Effect?
The bullwhip effect causes havoc in the supply chain. It’s triggered by small changes at the demand end of the chain, that are increasingly amplified upstream in the supply network – like the movement at the end of a bullwhip. The more remote a supply chain node is from the originating demand signal, the greater the exaggerated response.
“The bullwhip effect is a distribution channel phenomenon in which demand forecasts yield supply chain inefficiencies. It refers to increasing swings in inventory in response to shifts in consumer demand as one moves further up the supply chain.[2]”
The bullwhip effect can be contained by reducing information time lags and sources of variability in the supply chain, both demand variability and supply variability. This can be achieved by through sharing a real-time single version of the truth with all participants, so that all parties know actual demand and supply across the network at all times. This eliminates the need to estimate demand and orders, and hence eliminates the root cause of bullwhip effect.
The concept first appeared in Jay Forrester’s Industrial Dynamics (1961)[1] and thus it is also known as the “Forrester effect.” It has been described as “the observed propensity for material orders to be more variable than demand signals and for this variability to increase the further upstream a company is in a supply chain.[2]”
Resilience of the Network-Based Approach
As mentioned, One Network has deployed very large supply networks in the Food Retailing and Food Service sectors. As we entered the pandemic and demand began to shift, our customers easily managed the new uncertainties for themselves and their upstream distributors, suppliers, producers and farmers by turning a few dials in their network settings and leveraging the predictive and prescriptive analytics within our platform. This allowed them to react in real time, as well as adjust their planning across all time horizons by leveraging advanced insights regarding the “next normal” post-pandemic.
The statistical shift is real. The question is what are the right scenarios to model related to future consumer spending, and whether you have a demand and supply network technology infrastructure that will give you the flexibility to react in both the short and long term. Our NEO agent-based network and prescriptive analytics can do all the heavy lifting in this area and provide deep industry-based insights to help with your decision making.
In a recent McKinsey survey of the food and consumer-goods industries, 100% of respondents had experienced production and distribution problems, 91% had problems with suppliers and a whopping 85% of respondents struggled with inefficient digital technologies in their supply chains. They go on to state, “We believe that leaders should take this moment not just to fix their supply chains temporarily, but to transform them. Reimagining supply chains to avoid past traps and meet future needs will require a more comprehensive approach.”
Single Points of Failure & Disruption in the Meat Supply Chain
As we look more closely at the upstream disruption created in the protein supply network through the demand shift, we need to understand the evolution of the industry structure. A few decades ago, the meat supply network was fragmented with many small players operating packing plants (with cattle and hogs running at about 1,000 head per day, selling the animal in bulk). As the network became more sophisticated in terms of packaging and shipment, smaller operators did not have the wherewithal to make the required investments and so processing moved to large footprint operations running as many as 6,000 head per shift for cattle and 20,000 for hogs.
In supply network terms, this physical shift (that was done for cost reasons) created a single point of failure within certain geo-based subnetworks from a demand and supply perspective. If we look at Tyson for example, they were able to react to a plant closing due to a fire by running extra shifts at other plants. However, significant shutdowns across multiple nodes in the network, as experienced in the pandemic, led them to having to “depopulate” upstream supplies of poultry and pork. We also saw the dumping of milk and other items due to bulk packaging issues related to demand shifts from food service to retail. Fortunately, there is a program called the Farmers to Families Food Box Program where the federal government buys meat, produce and dairy from farmers to distribute to people in need. Estimates are that this would provide over 50 million meals through the summer, helping to reduce growing waste in the supply network.
There is a close parallel here for produce, especially fresh and ultra-fresh produce. The protein-based supply chain is finely tuned to harvest animals at a certain time, weight, or physical characteristic, and move the product (with the proper packaging and shipping, with real time tracking, and meeting cold chain and FDA requirements) to the end consumer. This is really no different than the planning and execution of the fruit and vegetable harvest, and the subsequent product movement downstream to the end consumer. (Another sector that could benefit from an end-to-end supply network infrastructure would be generic pharmaceuticals where there are significant geographic points of failure based on consolidation and outsourcing.)
Optimization in Fresh and Ultra-Fresh Produce
To improve performance in fresh and ultra-fresh produce, optimal inventory management depends on forecast accuracy, inventory carrying cost, product perishability, lost sales and inventory shrinkage costs. The “trade-off” between service levels and inventory costs is a key focal point for analysis, along with the impact of increased forecast accuracy on inventory-related costs. The point here is that there is no trade-off at all. You can improve service levels and lower inventory costs at the same time.
The results of process improvements enabled by a supply network platform thus far have been strong. In a recent case, the new process enabled the lowering of on-hand inventory levels at the DC and retail stores to 0.5 and 1.5 days, respectively, improving fresh-relevant costs by 31%. Optimizing inventory policies has improved item fill rate (IFR) at the DC from 91.7% to 95.2%, having a significant impact at the retail level in on-shelf availability, competitiveness, and lost sales to alternatives. Major cost improvements have been generated from reductions in inventory holding costs, inventory shrinkage costs, and waste reduction (spoilage). One Network’s ability to perform last minute allocation (LMA) allows for the planning and execution of order, delivery, and inventory policies at optimal cost levels while driving retail IFR up to 99%, achieving target service levels with high product quality at the least landed cost.
The Importance Supply Network Design
So how do we design our supply network for the “next normal” in the protein and produce industries? Concentration brings us cost advantages, but also comes with problems in business continuity, resiliency, and operational readiness. Highly distributed supply brings increased costs both from a fixed and variable perspective. So what’s best?
Fortunately, today we have the ability to fully model the network and run analytics around thousands of scenarios which will provide options, prescriptions and decision-making related to both the supply network structure as well as planning and execution. Today, poultry is nearly 100% vertically integrated and is therefore the most fragile network, followed by pork at just less than 50% and finally beef which is a little more fragmented – and thus more resilient to locational disruption. That’s the top down view, but the reality is that individual producers and retailers have many options and choices to evaluate in their supply network design.
The potential for a poorly designed networks really hit home for many consumers when Wendy’s had to drop burgers from their menus at almost 1,000 QSR’s due to meat shortages. However, this left an opening for plant-based protein burgers to fill the void showing growth rates of over 100%. Given that plant-based burger production is highly automated, these businesses did not suffer much of the worker-based disruption we saw in the traditional meat plants. We have been able to avoid significant price gouging as we have moved through the pandemic, but by no means does anyone now feel they have anywhere near the desired or required level of supply network functionality and resilience for the future.
Plan and Execute with Real-Time Information
As mentioned earlier, one of our food manufacturing customers had made dramatic improvements, reaching 99% in-store/in-stock levels including promoted items with only 25 days inventory in the network. This superior level of demand/supply match is even more important when considering perishables like protein and produce.
At One Network, we have large restaurant chains on the network along with their upstream trading partners which include distributors, meat packers, slaughter houses, ranchers and even the CBOT (Chicago Board of Trade) where the futures contracts for the beef are secured. Similar to the promotion demand accuracy and fulfillment problem in retail, food service and restaurants have a problem forecasting LTO’s (limited time offerings) along with forecasting base demand, given the many option levels in their standard menu items. The real key in both the retail and food service scenarios is (1) the application of machine learning in demand analysis which can generate a more accurate forecast and (2) combining this with the blending of planning and execution in real time. When planning and execution operate together with real-time information, business can react to any variance-to-plan and secure replenishment along with coordinating upstream supply to maintain 100% customer service levels at the least landed cost.
Regardless of how accurate we can be in our forecasting, variance and disruption will occur. For this reason, we need the ability to react in real time, employing capabilities like last minute allocation in order to best serve our customer needs at lowest possible cost. This is particularly important today, when the past is no guide to the future, and your best course is to maximize your ability to respond optimally – using the best analytics and real-time information at hand.
Trend: Turning to New Channels and the Direct-to-Consumer (D2C) Option
Many meat producers and packers have added a consumer-direct channel in order to offset falling demand in the food service channel. This brings new challenges in the areas of last mile delivery and multi-echelon inventory optimization (MEIO). They also have challenges around inventory supplies such as frozen meats, given that meat cannot be frozen twice and once thawed needs to move flawlessly across the network with perfect demand/supply matching in real time – even when recut and repackaged.
Structural changes to the supply network are also being considered by both food retailers and food manufacturers, given the need to add micro-fulfillment centers for D2C at retail locations, along with remodeling storage facilities for manufacturers based on costs related to cold chain.
Statistics show that prior to the pandemic only 3% of grocery retailer volume was e-commerce. This has now grown to as much as 20% causing a costly labor shift from the consumer picking their items at the grocery stores to the retailer providing this service. This may also manifest itself in the creation of additional cross docking network nodes to support last mile delivery.
For manufacturers, the capacity utilization performance targets of the existing storage assets were designed under a different demand/supply mix scenario based on the old food service demand profiles which continue to shift.
Trend: Healthier Options, Grass Fed, Free Range and Organic
The trend toward “more healthy” food consumption has implications for meat producers as well. Grass-fed beef does not run through a feedlot which has traditionally acted somewhat as an inventory buffer in the beef supply network, similar to a staging area in a warehouse. So, for grass fed beef, moving the product downstream through the supply network without a buffer requires a more perfect demand/supply match, similar to what we see in Produce. It also requires the ability to react to problems and disruptions in real time. This capability is essential in the food industries, whether it’s the beef supply chain, pork supply chain or the chicken supply chain.
Trend: Shifts in Global Demand Will Continue
For beef producers who export, countries like China are expected to represent 30% of all incremental beef demand over the next few years, necessitating advanced global demand-supply match capabilities, global ATP, and global logistics. The variables in play are considerable given the fluctuations in protein and produce quality, exchange rates, transportation and logistics costs.
Demand shifts will continue along with opportunities to take advantage of new demand generation across expanded downstream channels.
An end-to-end software platform designed to be demand-driven across both planning and execution that can operate in real time and leverage AI/ML analytics will maximize asset efficiency along with delivering on quality product and customer service levels at the lowest landed cost. Core to the analytics is the ability to consider trade policy, feed costs, resource constraints, and consumer preferences. These analytics feed a real-time architecture designed to understand and react to operational disruptions in order to maintain cost competitiveness regionally, nationally, and globally.
Platform Requirements to Optimize Supply Chain Performance
The software platform of the future must be able to deliver the following capabilities, given that we need to manage not only cost, quality, and customer service, but also provide continuity, resiliency, operational readiness, and manage demand and supply disruptions in real time.
- End-to-end visibility and collaboration across trading partners. This capability goes to the core of the network. It needs to span seamlessly across planning and execution, allowing for not only visibility, but to also make decisions actionable across trading partners.
- Real-time demand-supply match. Across all planning and execution time horizons, constantly synchronize supply and demand on a continuous, daily basis. Leverage access to all variables related to orders, transportation, capacity, inventory (including intransit inventory), network structure, alternative sourcing, and shift management. Smooth out unexpected peaks or disruptions to maximize service levels at lowest cost.
- Autonomous forecasting. While demand-to-supply match is typical of an S&OP cycle, companies need to extend that capability so that they are running continuous demand-supply match multiple times per day. Whether you are in food retailing or food service, protein or produce, you need the ability to react to variances during the day, during the week, during the month, and during the quarter.
- Automatic order generation, aggregation, and load building. Based on the results of autonomous forecast analytics, provide the ability to automatically create replenishment orders so that managers can focus on other tasks while simultaneously reducing order variance to demand. The focus needs to be on lowest landed cost while also improving customer service levels and reducing inventory carrying costs.
- Multi-pick/multi-drop load optimization. Efficiency and flexibility based on reacting in real time to retail or food service needs, while also optimizing for transportation costs and waste.
- Autonomous appointment scheduling. It’s all about flow, asset utilization, and employee productivity. Get the right product to the right door at the right time with minimal effort.
- Last minute allocation. Variance to plan, forecast errors, network problems, random disruptions will always occur. It is critical to reallocate based on real-time available supply to generate the best result around customer service.
- Last mile delivery. Channels are shifting and this capability is required to address new demand opportunities.
- Order expediting. Whether it is a projected stockout or some other type of disruption that will affect customer service or an OTIF (on-time, in-full) delivery, quickly evaluating prescriptions related to order management and acting on those recommendations is core.
- Tracking, cold chain, and network event management. Tracking, including serialization (along with various forms of product safety and related driver procedures) have really taken center stage during the COVID crisis. Food safety cold chain event management, tracking, and reporting along with IoT integration have become ever more important. Events can include promotions, LTO’s, vendor tracking, carrier tracking and more. Understanding and planning for milestone management along with prescriptions to react to events when things go wrong is key. You’ll also need to be able to measure supplier performance in these areas.
- AI/machine learning. The “next normal” will require us to analyze an expanded set of data/vectors/influences on serving end-consumer demand. Machine learning is designed specifically to address this need, along with Deep Learning/Neural Nets for pattern recognition.
- Multi-echelon inventory optimization. MEIO is a core capability required for an efficient demand-supply match while keeping a focus on customer service levels and lowest landed cost. The policies will reflect risk levels related to demand, inventory, logistics, capacity, forecast variance and other variables in order to set the correct rhythm and cadence for the network overall.
- Network node expansion or consolidation. The ability to enable pop-up warehouses, slow moving warehouses, or micro fulfillment centers as part of the network is evolving quickly. So is the need to consolidate in areas like cold chain where volumes have dropped in food service. The need is for rapid order promising, fulfillment, and allocation from simple stocking locations or container ships, along with additional network nodes. For example, this ensures that retail locations aren’t over-sourced to provide for home delivery.
- Planning, simulation, and scenario analysis. Data is where everything starts, and without a detailed network representation the data really isn’t very useful. A demand-driven real-time network is required, one whose representation starts at the item level and builds through demand, supply, logistics and fulfillment. It should also provide AI/ML analytics around prescriptive decision making across all time horizons. By having all nodes in the supply network represented, the ability to ensure asset optimization, multi-echelon inventory optimization, and order delivery on-time in-full is ensured.
- Digital twin. A digital twin needs to be an extension of the actual planning and execution environment where prescriptive analytics can be evaluated to react to problems and optimize opportunities. This provides the important advantage of being able to execute those decisions in one seamless flow.
- Alternate sourcing. The network intelligence embodied in the autonomous agents needs to run thousands of scenarios in the background once the network is instantiated. Risk analysis is a big part of the activity and as part of that analysis alternate sourcing candidates along with the timing to shift sourcing based on increasing risk factors. Optimization through MEIO is a key contributor to this level of analysis.
Sector Concerns and the Need for Multi-Party Collaboration
As we look across our supply network, we find pressures shift depending on position within the network, but that all trading partners need to work collaboratively through multi-party transactions in order to generate results beneficial to all.
Farmers and Ranchers: Fresh produce is labor intensive, so any type of labor restriction needs to be considered. Many decisions are annual in nature from a planning perspective, but do involve big decisions in terms of crop selection and volumes. For beef, dairy and sometimes poultry, the recovery cycle for planned pivots in supply typically takes years.
Food Service and Restaurant Distributors: Steady flow is what it is all about. Real-time demand-supply match is the name of the game, and it needs to be near perfect. When there are disruptions to outbound orders, delays in rebalancing with upstream shipments fulfilling inbound supply can be very costly and will quickly consume available storage space creating bottlenecks across the network. Moving forward, restaurant and food service channels will continue to operate on lower volumes and so cold chain storage facilities will be underutilized. There are multiple scenarios available to improve margins in these situations.
Food Service and Restaurant Producers: Similar to the distributors, volumes have dropped and retail isn’t quite large enough to make up the difference given the packaging differences and related investments to operationalize those channels. Thus, we have an asset utilization problem. Key will be to analyze and deploy scenarios to achieve breakeven volumes and beyond, same for the distributors.
Food Manufacturers and Meat Packing: Distribution challenges into retail grocery are the current focal point, given the surge in demand for this channel. These are magnified by the lack of a software network which can provide a coordinated and simultaneous real-time capability for managing orders together with logistics and inventory. Increased single-serve packaging for the retail channel as the food service channel demand decreases is also an issue for both production and logistics.
Grocery and Food Retailers: The growth in demand and the shift away from restaurants and food service is very real and causing multiple logistical issues for retailers, including physical shelf space and inventory management along with the on-line volumes and the related replenishment and last mile delivery challenges. While volumes have soared in this channel, margin quality has dropped. The cost to service these expanded channels along with the expanded volumes was not something their current software systems and platforms were designed to support. For the most part those systems are hard-wired, and any change or need for flexibility is poorly supported at best. Shifting to a modern architecture would solve these issues and not only bring margins back to previous levels, but certainly exceed them.
Restaurants and Food Service Businesses: While this space is down right now, alternate channels are rapidly evolving for pick up and home delivery. The traditional challenges of poor sales history, forecasts and promotional accuracy will now be compounded significantly. Menu translation into material requirements still remains a problem, along with a lack of reference data.
In Summary
In the McKinsey report referenced earlier, the analysts go on to say, “Boosting the supply chain’s end-to-end digital capabilities requires a coordinated view across nodes so that companies can connect the dots with the latest digital tools and capabilities. Autonomous planning systems with machine-learning capabilities can base their forecasts on many more factors and learn the “next normal” much faster than traditional approaches for building business continuity, preserving cash, and strengthening supply-chain resilience.” We certainly agree.
2020 has been a year like no other for the protein and produce supply chains, with shifts in demand, shifts in supply, and changes in consumer behavior – many of which will likely remain permanent. We believe that business network technology will help maximize your success in this new environment. When planning and execution operate together with real-time information and advanced analytics, businesses are best positioned react to any variance and secure replenishment, while coordinating upstream supply for the highest customer service levels at the least landed cost.